The Importance of Bounding Boxes in Data Annotation
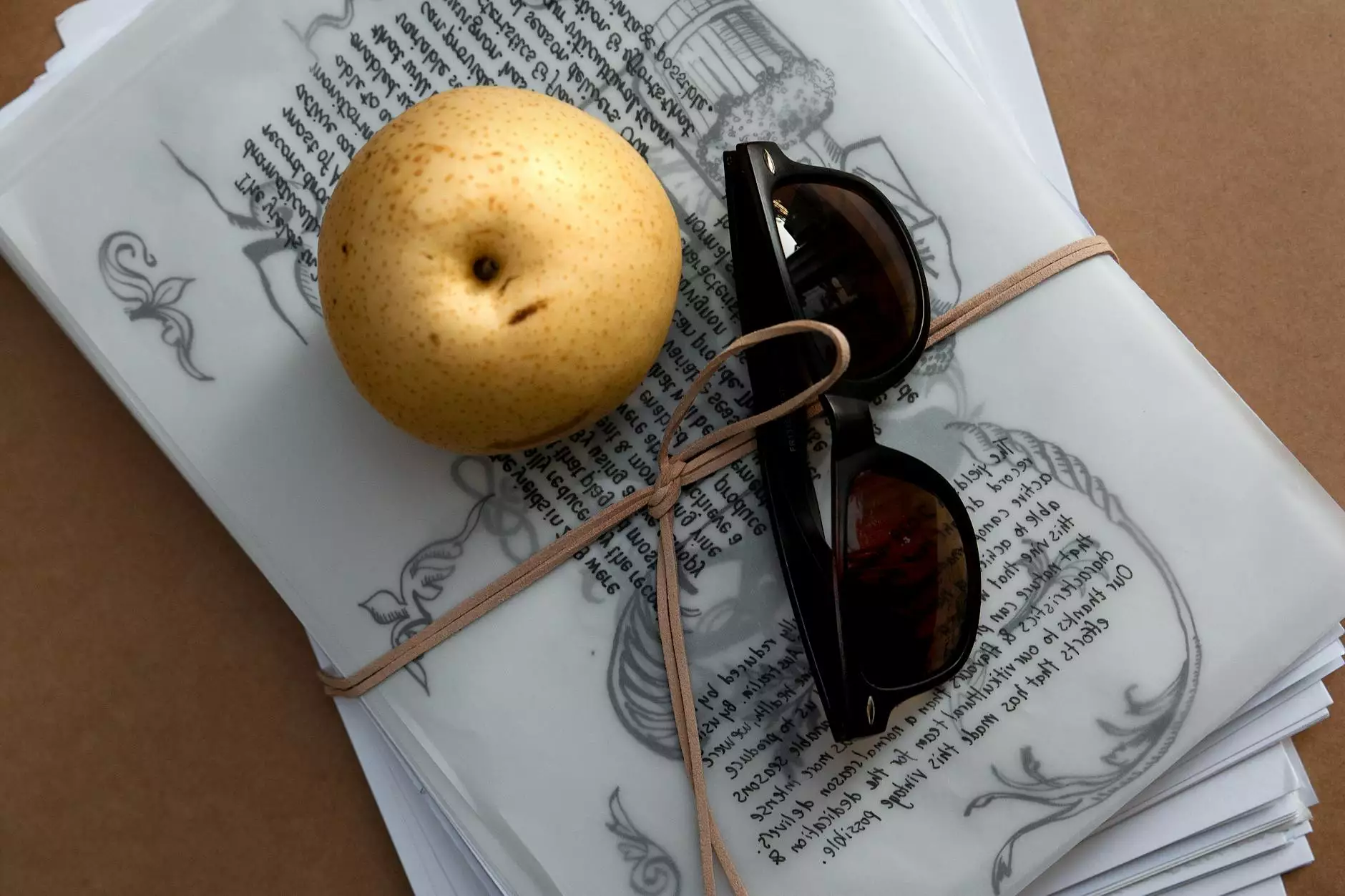
In the ever-evolving landscape of artificial intelligence (AI) and machine learning (ML), the role of data annotation cannot be overstated. One of the fundamental techniques utilized in this process is bounding boxes. This article delves into what bounding boxes are, their applications, and their pivotal role in enhancing machine learning models, particularly in the context of a sophisticated solution like KeyLabs.ai.
Understanding Bounding Boxes
Bounding boxes are rectangular borders that are often used in image processing and computer vision applications to define the location of objects within a visual field. These boxes are crucial for tasks such as:
- Object Detection: Identifying and locating objects in images.
- Image Segmentation: Distinguishing objects from the background.
- Tracking: Following the movement of objects across frames in a video.
The Mechanism of Bounding Boxes
When a bounding box is created, it is represented by coordinates (typically x, y coordinates for the top-left corner along with width and height). This offers a comprehensive method for marking an object’s extent in an image. The bounding box provides a simplified representation of complex shapes and objects, making it easier for algorithms to process and learn from visual data.
Applications of Bounding Boxes
Bounding boxes see a wide range of applications across various sectors. Here are some notable examples:
1. Autonomous Vehicles
In the field of autonomous driving, bounding boxes are essential for detecting pedestrians, vehicles, road signs, and more. They help the vehicle’s AI understand its environment and make informed decisions for navigation.
2. Retail and Inventory Management
Retail AI uses bounding boxes for automated inventory management. By recognizing product placement and stock levels through image recognition, businesses can optimize storage and reduce shrinkage.
3. Medical Imaging
In healthcare, bounding boxes are used to identify tumors or other anomalies in radiology images. This assists radiologists by highlighting areas that require further examination.
4. Augmented Reality (AR)
Bounding boxes play a critical role in AR applications by allowing virtual objects to be anchored to real-world locations, enhancing user interaction and experience.
The Benefits of Using Bounding Boxes in Data Annotation
Incorporating bounding boxes into the data annotation process offers several advantages, including:
- Ease of Use: Creating bounding boxes is user-friendly, allowing annotators to quickly identify and tag objects in images.
- Improved Accuracy: Accurate bounding boxes lead to better training datasets, which in turn yield more reliable models.
- Scalability: Tools like KeyLabs.ai support the parallel processing of bounding boxes, enabling the rapid annotation of large datasets.
KeyLabs.ai: A Leading Data Annotation Platform
KeyLabs.ai stands out as a top-tier data annotation platform that offers comprehensive solutions for creating high-quality bounding boxes efficiently. The platform is designed with advanced features tailored for a variety of industries:
1. User-Friendly Interface
The intuitive interface of KeyLabs.ai allows users to create and edit bouding boxes effortlessly. This streamlines the data annotation workflow, ensuring that teams can focus on quality and output rather than grappling with complicated software.
2. Robust Quality Assurance Mechanisms
KeyLabs.ai integrates multiple layers of quality control to ensure that the bounding boxes created in the data annotation process are accurate and reliable. Their system facilitates collaborative reviews and feedback loops to maintain the highest standards.
3. Scalability and Flexibility
Whether you are processing thousands of images or millions, KeyLabs.ai adapts to your workload with its scalable infrastructure. This means organizations can effortlessly handle growing datasets without compromising on speed or quality.
Implementing Bounding Boxes: Best Practices
To maximize the effectiveness of bounding boxes in your projects, consider employing these best practices:
- Define Clear Guidelines: Establish comprehensive documentation on how bounding boxes should be created to maintain consistency across your dataset.
- Utilize Quality Control: Implement a robust review process to catch errors during the annotation phase.
- Train Your Annotators: Continuous training ensures that your team is proficient in using tools to create accurate bounding boxes.
Conclusion
Bounding boxes are an integral component of modern data annotation techniques. Their ability to enhance object recognition and provide clarity in complex visual datasets makes them indispensable in domains ranging from autonomous vehicles to medical imaging. Platforms such as KeyLabs.ai not only simplify this process but also ensure high levels of accuracy and efficiency. By embracing bounding boxes in your data annotation strategy, you ensure that your machine learning models are built on a strong and reliable foundation, paving the way for success in the competitive landscape of AI.
As our reliance on AI grows, understanding and utilizing effective tools like bounding boxes will continue to play a crucial role in driving innovation and accuracy across various sectors. By focusing on quality and leveraging platforms like KeyLabs.ai, businesses can stay ahead of the curve and effectively harness the power of data-driven solutions.